
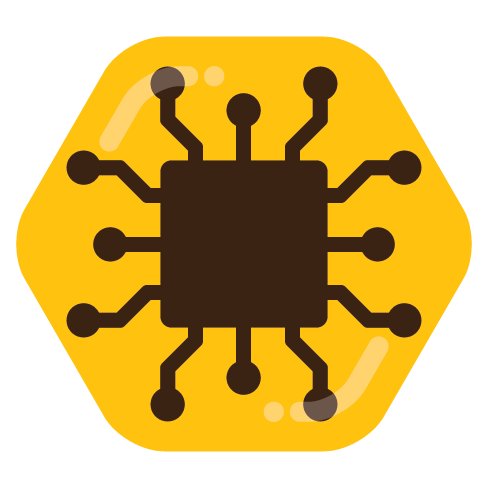
Deep learning has always been classified as AI. Some consider pathfinding algorithms to be AI. AI is a broad category.
AGI is the acronym you’re looking for.
Deep learning has always been classified as AI. Some consider pathfinding algorithms to be AI. AI is a broad category.
AGI is the acronym you’re looking for.
This feels to me like the LLM misinterpreted it as some kind of fictional villain talk and started to autocomplete it.
Could also be the model simply breaking. There was a time when Sydney (Bing AI or whatever they call it now) had to be constrained to 10 messages per context and having some sort of supervisor on top of itself because it would occasionally throw a fit or start threatening the user for no reason.
The author’s suggesting that smart people are more likely to fall for cons that they try to dissect but can’t find the specific method being used, supposedly because they consider themselves to be infallible.
I disagree with this take. I don’t see how that thought process is exclusive to people who are or consider themselves to be smart. I think the author is tying himself into a knot to state that smart people are actually the dumb ones, likely in preparation to drop an opinion that most experts in the field will disagree with.
Github link for convenience
https://github.com/Anuken/Mindustry
GPT3 is pretty bad at it compared to alternatives (although it’s hard to compete with calculators on that field), but if it was just repeating after the training dataset it would be way worse. From the study I’ve linked in my other comment (https://arxiv.org/pdf/2005.14165.pdf):
On addition and subtraction, GPT-3 displays strong proficiency when the number of digits is small, achieving 100% accuracy on 2 digit addition, 98.9% at 2 digit subtraction, 80.2% at 3 digit addition, and 94.2% at 3-digit subtraction. Performance decreases as the number of digits increases, but GPT-3 still achieves 25-26% accuracy on four digit operations and 9-10% accuracy on five digit operations, suggesting at least some capacity to generalize to larger numbers of digits.
To spot-check whether the model is simply memorizing specific arithmetic problems, we took the 3-digit arithmetic problems in our test set and searched for them in our training data in both the forms " + =" and " plus ". Out of 2,000 addition problems we found only 17 matches (0.8%) and out of 2,000 subtraction problems we found only 2 matches (0.1%), suggesting that only a trivial fraction of the correct answers could have been memorized. In addition, inspection of incorrect answers reveals that the model often makes mistakes such as not carrying a “1”, suggesting it is actually attempting to perform the relevant computation rather than memorizing a table.
In my comment I’ve been referencing https://arxiv.org/pdf/2005.14165.pdf, specifically section 3.9.1 where they summarize results of the arithmetic tasks.
That’s not entirely true.
LLMs are trained to predict next word given context, yes. But in order to do that, they develop internal model that minimizes error across wide range of contexts - and emergent feature of this process is that the model DOES perform more than pure compression of the training data.
For example, GPT-3 is able to calculate addition and subtraction problems that didn’t appear in the training dataset. This would suggest that the model learned how to perform addition and subtraction, likely because it was easier or more efficient than storing all of the examples from the training data separately.
This is a simple to measure example, but it’s enough to suggests that LLMs are able to extrapolate from the training data and perform more than just stitch relevant parts of the dataset together.
They can replace them going forward. A major issue is that many governments (and likely other malicious actors) have been hoarding encrypted communication in hopes of accessing it once sufficiently big quantum computer emerges.
Was this ever a thing? I have never seen or heard anyone use “gen AI” to mean AGI. In fact I can’t even find one instance of such usage.